skactiveml.pool.QueryByCommittee#
- class skactiveml.pool.QueryByCommittee(method='KL_divergence', eps=1e-07, sample_predictions_method_name=None, sample_predictions_dict=None, missing_label=nan, random_state=None)[source]#
Bases:
SingleAnnotatorPoolQueryStrategy
Query-by-Committee (QBC)
The Query-by-Committee (QBC) strategy uses an ensemble of estimators to identify on which samples many estimators disagree.
- Parameters
- method“KL_divergence” or “vote_entropy” or “variation_ratios, default=’KL_divergence’
The method to calculate the disagreement in the case of classification. ‘KL_divergence’, ‘vote_entropy’, and ‘variation_ratios’ are possible. In the case of regression, this parameter is ignored and the empirical variance is used.
- epsfloat > 0, default=1e-7
Minimum probability threshold to compute log-probabilities (only relevant for method=’KL_divergence’).
- sample_predictions_method_namestr, default=None
Certain estimators may offer methods enabling to construct a committee by sampling predictions of committee members. This parameter is to indicate the name of such a method.
If sample_predictions_method_name=None no sampling is performed.
If sample_predictions_method_name is not None and in the case of classification, the method is expected to take samples of the shape (n_samples, *) as input and to output probabilities of the shape (n_members, n_samples, n_classes), e.g., sample_proba in skactiveml.base.ClassFrequencyEstimator.
If sample_predictions_method_name is not None and in the case of regression, the method is expected to take samples of the shape (n_samples, *) as input and to output numerical values of the shape (n_members, n_samples), e.g., sample_y in sklearn.gaussian_process.GaussianProcessRegressor.
- sample_predictions_dictdict, default=None
Parameters (excluding the samples) that are passed to the method with the name sample_predictions_method_name.
This parameter must be None, if sample_predictions_method_name is None.
Otherwise, it may be used to define the number of sampled members, e.g., by defining n_samples as parameter to the method sample_proba of skactiveml.base.ClassFrequencyEstimator or sample_y of sklearn.gaussian_process.GaussianProcessRegressor.
- missing_labelscalar or string or np.nan or None, default=np.nan
Value to represent a missing label.
- random_stateint or np.random.RandomState or None, default=None
The random state to use.
References
- 1
H.S. Seung, M. Opper, and H. Sompolinsky. Query by committee. In ACM Workshop on Computational Learning Theory, pages 287-294, 1992.
- 2
N. Abe and H. Mamitsuka. Query Learning Strategies Using Boosting and Bagging. In International Conference on Machine Learning, pages 1-9, 1998.
- 3
Burbidge, Robert and Rowland, Jem J and King, Ross D. Active Learning for Regression Based on Query by Committee. In International Conference on Intelligent Data Engineering and Automated Learning, pages 209-218, 2007.
- 4
Beluch, W. H., Genewein, T., Nürnberger, A., and Köhler, J. M. The Power of Ensembles for Active Learning in Image Classification. In Conference on Computer Vision and Pattern Recognition, pages 9368-9377, 2018
Methods
Get metadata routing of this object.
get_params
([deep])Get parameters for this estimator.
query
(X, y, ensemble[, fit_ensemble, ...])Determines for which candidate samples labels are to be queried.
set_params
(**params)Set the parameters of this estimator.
- get_metadata_routing()#
Get metadata routing of this object.
Please check User Guide on how the routing mechanism works.
- Returns
- routingMetadataRequest
A
MetadataRequest
encapsulating routing information.
- get_params(deep=True)#
Get parameters for this estimator.
- Parameters
- deepbool, default=True
If True, will return the parameters for this estimator and contained subobjects that are estimators.
- Returns
- paramsdict
Parameter names mapped to their values.
- query(X, y, ensemble, fit_ensemble=True, sample_weight=None, candidates=None, batch_size=1, return_utilities=False)[source]#
Determines for which candidate samples labels are to be queried.
- Parameters
- Xarray-like of shape (n_samples, n_features)
Training data set, usually complete, i.e. including the labeled and unlabeled samples.
- yarray-like of shape (n_samples,)
Labels of the training data set (possibly including unlabeled ones indicated by self.missing_label.)
- ensemblearray-like of SkactivemlClassifier or array-like of SkactivemlRegressor or SkactivemlClassifier or SkactivemlRegressor
If ensemble is a SkactivemlClassifier or a SkactivemlRegressor and has n_estimators plus estimators_ after fitting as attributes, its estimators will be used as committee.
If ensemble is array-like, each element of this list must be SkactivemlClassifier or a SkactivemlRegressor and will be used as committee member.
If ensemble is a SkactivemlClassifier or a SkactivemlRegressor and implements a method with the name sample_predictions_method_name, this method is used to sample predictions of committee members.
- fit_ensemblebool, default=True
Defines whether the ensemble should be fitted on X, y, and sample_weight.
- sample_weight: array-like of shape (n_samples,), default=None
Weights of training samples in X.
- candidatesNone or array-like of shape (n_candidates), dtype=int or array-like of shape (n_candidates, n_features), default=None
If candidates is None, the unlabeled samples from (X,y) are considered as candidates.
If candidates is of shape (n_candidates,) and of type int, candidates is considered as the indices of the samples in (X,y).
If candidates is of shape (n_candidates, *), the candidate samples are directly given in candidates (not necessarily contained in X). This is not supported by all query strategies.
- batch_sizeint, default=1
The number of samples to be selected in one AL cycle.
- return_utilitiesbool, default=False
If true, also return the utilities based on the query strategy.
- Returns
- query_indicesnumpy.ndarray of shape (batch_size)
The query_indices indicate for which candidate sample a label is to queried, e.g., query_indices[0] indicates the first selected sample.
If candidates is None or of shape (n_candidates,), the indexing refers to the samples in X.
If candidates is of shape (n_candidates, n_features), the indexing refers to the samples in candidates.
- utilitiesnumpy.ndarray of shape (batch_size, n_samples) or numpy.ndarray of shape (batch_size, n_candidates)
The utilities of samples after each selected sample of the batch, e.g., utilities[0] indicates the utilities used for selecting the first sample (with index query_indices[0]) of the batch. Utilities for labeled samples will be set to np.nan.
If candidates is None or of shape (n_candidates,), the indexing refers to the samples in X.
If candidates is of shape (n_candidates, n_features), the indexing refers to the samples in candidates.
- set_params(**params)#
Set the parameters of this estimator.
The method works on simple estimators as well as on nested objects (such as
Pipeline
). The latter have parameters of the form<component>__<parameter>
so that it’s possible to update each component of a nested object.- Parameters
- **paramsdict
Estimator parameters.
- Returns
- selfestimator instance
Estimator instance.
Examples using skactiveml.pool.QueryByCommittee
#
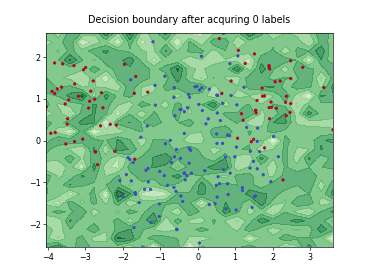
Query-by-Committee (QBC) with Kullback-Leibler Divergence
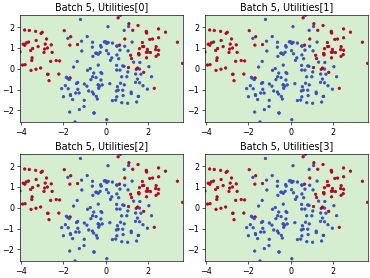
Batch Bayesian Active Learning by Disagreement (BatchBALD)