skactiveml.base.QueryStrategy#
- class skactiveml.base.QueryStrategy(random_state=None)[source]#
Bases:
ABC
,BaseEstimator
Base class for all query strategies in scikit-activeml.
- Parameters
- random_stateint or RandomState instance, optional (default=None)
Controls the randomness of the estimator.
Methods
Get metadata routing of this object.
get_params
([deep])Get parameters for this estimator.
query
(*args, **kwargs)Determines the query for active learning based on input arguments.
set_params
(**params)Set the parameters of this estimator.
- get_metadata_routing()#
Get metadata routing of this object.
Please check User Guide on how the routing mechanism works.
- Returns
- routingMetadataRequest
A
MetadataRequest
encapsulating routing information.
- get_params(deep=True)#
Get parameters for this estimator.
- Parameters
- deepbool, default=True
If True, will return the parameters for this estimator and contained subobjects that are estimators.
- Returns
- paramsdict
Parameter names mapped to their values.
- abstract query(*args, **kwargs)[source]#
Determines the query for active learning based on input arguments.
- set_params(**params)#
Set the parameters of this estimator.
The method works on simple estimators as well as on nested objects (such as
Pipeline
). The latter have parameters of the form<component>__<parameter>
so that it’s possible to update each component of a nested object.- Parameters
- **paramsdict
Estimator parameters.
- Returns
- selfestimator instance
Estimator instance.
Examples using skactiveml.base.QueryStrategy
#

Batch Active Learning by Diverse Gradient Embedding (BADGE)
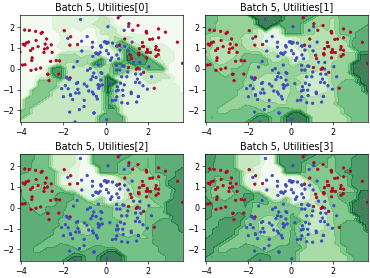
Batch Density-Diversity-Distribution-Distance Sampling
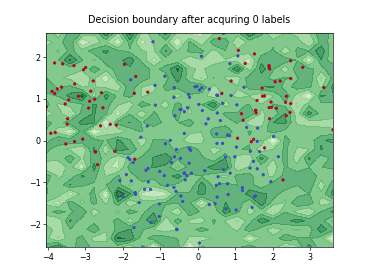
Query-by-Committee (QBC) with Kullback-Leibler Divergence
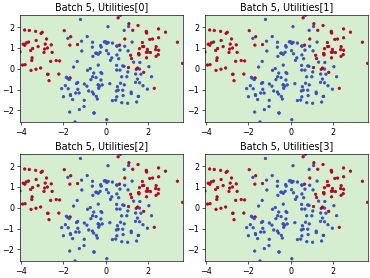
Batch Bayesian Active Learning by Disagreement (BatchBALD)

Regression Tree Based Active Learning (RT-AL) with Random Selection

Regression Tree Based Active Learning (RT-AL) with Diversity Selection

Regression Tree Based Active Learning (RT-AL) with Representativity Selection
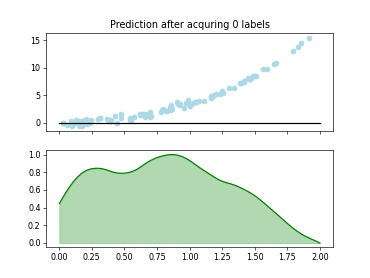
Regression based Kullback Leibler Divergence Maximization
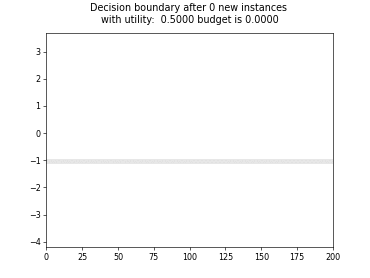
Cognitive Dual-Query Strategy with Random Sampling
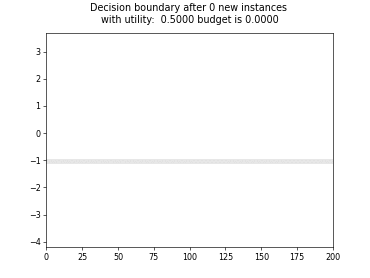
Cognitive Dual-Query Strategy with Fixed-Uncertainty
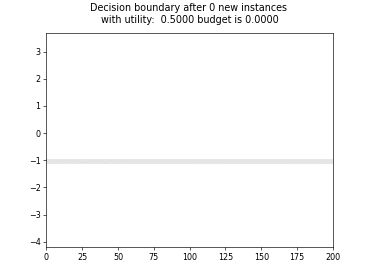
Cognitive Dual-Query Strategy with Variable-Uncertainty
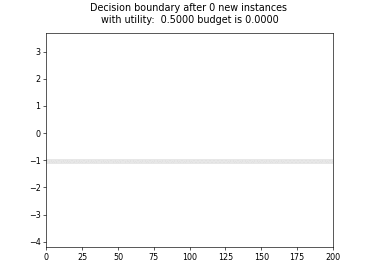
Cognitive Dual-Query Strategy with Randomized-Variable-Uncertainty